Table of Contents
fastsurfer_inference
is a ChRIS app that efficiently performs cortical parcellation and anatomical segmentation on raw brain MRI images. In actuality, the ChRIS app is wrapper/vehicle around the FastSurfer engine developed by the Deep Medical Imaging lab. This plugin only wraps around the neural network (responsible for image segmentation). FastSurfer also has a recon-surf surface pipeline.
This plugin is GPU-capable. In anecdotal testing, a full segmentation on the GPU takes in the order of a minute (or less). The same segmentation on CPU can take 90 minutes. Note for CPU running, a machine with high RAM is required. While not fully tested, we recommend at least 24GB RAM for CPU runs (although 16GB RAM might work).
For full information about the underlying method, consult the FastSurfer publication:
Henschel L, Conjeti S, Estrada S, Diers K, Fischl B, Reuter M. "FastSurfer - A fast and accurate deep learning based neuroimaging pipeline." NeuroImage. 2020.
Paper: http://dx.doi.org/10.1016/j.neuroimage.2020.117012 Bib: https://deep-mi.org/static/pub/henschel_2020.bib
The source code of FastSurfer is available on Github: https://github.com/Deep-MI/FastSurfer.
python fastsurfer_inference.py \ [--subjectDir <subjectDir>] \ [--subject <subjectToProcess>] \ [--in_name <inputFileToProcess>] \ [--out_name <segmentedFile] \ [--order <interpolation>] \ [--log <logFile>] \ [--clean] \ [--no_cuda] \ [--batch_size <batchSizePerInference] \ [--simple_run] \ [--run_parallel] \ [--copyInputFiles <searchKeyword>] \ [-v <level>] [--verbosity <level>] \ [--version] \ [--man] \ [--meta] \ <inputDir> <outputDir>
fastsurfer_inference.py
is a ChRIS-based application that is capable of whole brain segmentation into 95 classes.
Simply pull the docker image,
docker pull fnndsc/pl-fastsurfer_inference
and go straight to the examples section.
[--subjectDir <subjectDir>] By default, the <subjectDir> is assumed to be the <inputDir>. However, the <subjectDir> can be nested relative to the <inputDir>, and can thus be specified with this flag. The <subjectDir> is assumed by default to contain one level of sub directory, and these sub dirs, considered the ``subjects``, each contain a single ``mgz`` to process. [--subject <subjectToProcess>] This can denote a sub-set of subject(s) (i.e. sub directory within the <subjectDir>). The <subjectToProcess> is "globbed", so an expression like ``--subject 10*`` would process all ``subjects`` starting with the text string ``10``. Note to protect from shell expansion of wildcard characters, the argument should be protected in single quotes. [--in_name <inputFileToProcess>] The name of the raw ``.mgz`` file of a subject. The default value is ``brain.mgz``. The full path to the <inputFileToProcess> is constructed by concatenating ``<inputDir>/<subjectDir>/<subject>/<inputFileToProcess>`` [--out_name <segmentedFile] The name of the output or segmented ``mgz`` file. Default name is ``aparc.DKTatlas+aseg.deep.mgz`` [--order <interpolation>] The order of interpolation: 0 = nearest 1 = linear (default) 2 = quadratic 3 = cubic [--log <logFile>] The name of the log file containing inference info. Default value is ``deep-seg.log`` [--clean] If specified, clean the segmentation. [--no_cuda] If specified, run on CPU, not GPU. Depending on CPU/GPU, your apparent mileage will vary, but expect orders longer time than compared to a GPU. For example, in informal testing, GPU takes about a minute per subject, while CPU approximately 1.5 hours per subject! [--batch_size <batchSizePerInference] Batch size per inference. Default is 8. [--simple_run] Simplified run: only analyse one given image specified by ``--in_name`` (output: ``--out_name``). Note that you need to specify absolute path to both ``--in_name`` and ``--out_name`` if this option is chosen. [--run_parallel] If multiple GPUs are present to the docker container, enable parallel computation on multiple GPUs with an inference run. [--copyInputFiles <searchKeyword>] If specified, copies the input file(s) matching the keyword to output dir. This can be useful to create an easy association between a given input file(s) and the segmented output. [-v <level>] [--verbosity <level>] Verbosity level for app. Not used currently. [--version] If specified, print version number. [--man] If specified, print (this) man page. [--meta] If specified, print plugin meta data.
The execute vector of this pluing is via docker
.
To run using docker
, be sure to assign an "input" directory to /incoming
and an output directory to /outgoing
. Make sure that the $(pwd)/out
directory is world writable!
Now, prefix all calls with
docker run --rm -v $(pwd)/out:/outgoing \
fnndsc/pl-fastsurfer_inference \
fastsurfer_inference \
Thus, getting inline help is:
mkdir in out && chmod 777 out
docker run --rm -v $(pwd)/in:/incoming -v $(pwd)/out:/outgoing \
fnndsc/pl-fastsurfer_inference \
fastsurfer_inference \
--man \
/incoming /outgoing
Assuming that the <inputDir>
layout conforms to
<inputDir>
│
└──<subjectDir>
│
├──<subject1>
│ │
│ └──█ brain.mgz
├──<subject2>
│ │
│ └──█ brain.mgz
├──<subject3>
│ │
│ └──█ brain.mgz
╎ ┄
╎ ┄
└──<subjectN>
│
└──█ brain.mgz
to process this (by default on a GPU) do
docker run --rm --gpus all \
-v $(pwd)/in:/incoming -v $(pwd)/out:/outgoing \
fnndsc/pl-fastsurfer_inference fastsurfer_inference \
/incoming /outgoing
(note the --gpus all
is not necessarily required) which will create in the <outputDir>
:
<outputDir>
│
└──<subjectDir>
│
├──<subject1>
│ │
│ └──█ aparc.DKTatlas+aseg.deep.mgz
├──<subject2>
│ │
│ └──█ aparc.DKTatlas+aseg.deep.mgz
├──<subject3>
│ │
│ └──█ aparc.DKTatlas+aseg.deep.mgz
╎ ┄
╎ ┄
└──<subjectN>
│
└──█ aparc.DKTatlas+aseg.deep.mgz
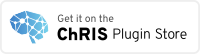