-
Notifications
You must be signed in to change notification settings - Fork 2
Commit
This commit does not belong to any branch on this repository, and may belong to a fork outside of the repository.
- Loading branch information
1 parent
699f808
commit 1079ef4
Showing
1 changed file
with
29 additions
and
0 deletions.
There are no files selected for viewing
This file contains bidirectional Unicode text that may be interpreted or compiled differently than what appears below. To review, open the file in an editor that reveals hidden Unicode characters.
Learn more about bidirectional Unicode characters
Original file line number | Diff line number | Diff line change |
---|---|---|
@@ -1 +1,30 @@ | ||
# Seg2Map :mag_right: :milky_way: | ||
|
||
_An interactive web map app for applying Doodleverse/Zoo models to geospatial imagery_ | ||
|
||
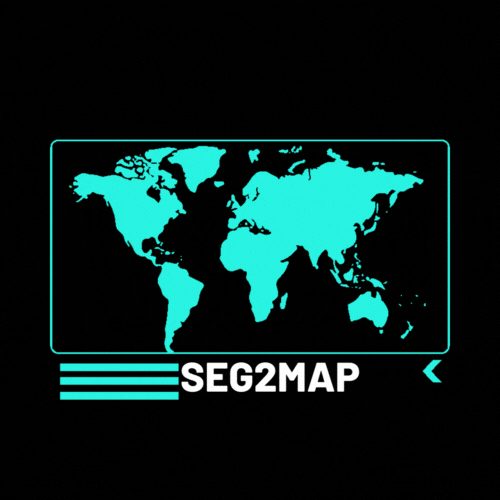 | ||
|
||
 | ||
|
||
## Overview: | ||
|
||
- Seg2Map facilitates application of Deep Learning-based image segmentation models and apply them to high-resolution (~1m or less spatial footprint) geospatial imagery, in order to make high-resolution label maps. Please see our [wiki](https://github.com/Doodleverse/seg2map/wiki) for more information. | ||
|
||
- The principle aim is to generate time-series of label maps from a time-series of imagery, in order to detect and assess land use/cover change. This project also demonstrates how to apply generic models for land-use/cover on publicly available high-resolution imagery at arbitrary locations. | ||
|
||
- Imagery comes from Google Earth Engine via [s2m_engine](https://github.com/Doodleverse/s2m_engine). Initially, we focus on [NAIP](https://www.usgs.gov/centers/eros/science/usgs-eros-archive-aerial-photography-national-agriculture-imagery-program-naip) time-series, available for the conterminious United States since 2003. In the future, [Planetscope](https://developers.planet.com/docs/data/planetscope/) imagery may also be made available (for those with access, such as federal researchers). | ||
|
||
- We offer a set of [Segmentation Zoo](https://github.com/Doodleverse/segmentation_zoo) models, especially created and curated for this project based on a publicly available datasets. These datasets have been selected because they are public, large (several hundred to several thousand labeled images), and provide broad class labels for generic land use/cover mapping needs. | ||
|
||
# Installation Instructions | ||
|
||
In order to use seg2map you need to install Python packages in an environment. We recommend you use [Anaconda](https://www.anaconda.com/products/distribution) to install the python packages in an environment for seg2map. After you install Anaconda on your PC, open the Anaconda prompt or Terminal in Mac and Linux and use the `cd` command (change directory) to go the folder where you have downloaded the seg2map repository. | ||
|
||
1. Create an Anaconda environment | ||
|
||
- This command creates an anaconda environment named `seg2map` and installs `python 3.10` in it | ||
- You can also use `python 3.10` | ||
- We will install the seg2map package and its dependencies in this environment. | ||
```bash | ||
conda create --name seg2map python=3.10 -y | ||
|